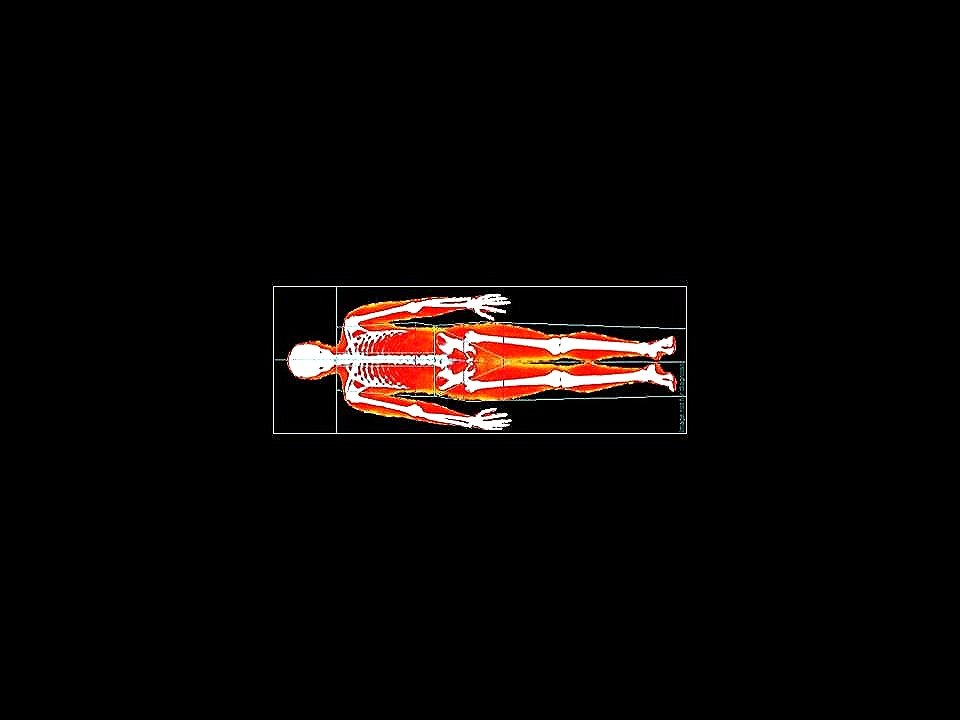
Research
The brief on Ben’s research
The majority of Ben’s research is at the intersection of using biomedical imaging to predict health, statistics, and the occasional machine learning project. Ben compared multiple biomedical imaging modalities, like MRI, ultrasound, and X-rays, to measure muscle health and how muscle health impacts survival and aging. Ben started this work during his PhD program in the lab of Adam Kuchnia and continues the research theme as a postdoctoral researcher with John Garrett. Ben also spent time as a postdoctoral researcher in the lab of Claudia Solis-Lemus developing a website focused on statistics and data science for middle and high schoolers. He brings his empathetic and open perspective to his research to improve its impact.
Ultrasound echo intensity correlated with MRI percent fat with & without correction
We explored the effectiveness of using ultrasound echo intensity (EI) to measure myosteatosis, a condition characterized by fat infiltration in muscles. By comparing raw and corrected EI with MRI-based proton density fat fraction (PDFF) across different forces from the ultrasound probe, we found that raw EI had a strong correlation with PDFF. Correction factors for subcutaneous fat thickness did not significantly enhance this relationship. These findings suggest that raw EI could serve as a practical, cost-effective method for assessing muscle health in clinical settings, especially for aging adults and those undergoing cancer treatment.
MRI vs Ultrasound vs CT
This study explores new biomarkers for fat infiltration in muscle, or myosteatosis, in sarcopenia and cachexia, comparing ultrasound and MRI techniques in different age groups, including lung cancer patients. It finds that proton density fat-fraction (PDFF) from MRI is a sensitive marker for myosteatosis across age groups. Echo intensity (EI) from ultrasound, while less sensitive, strongly correlates with PDFF. The study suggests that ultrasound could be a useful bedside tool for diagnosing muscle health issues, offering a more informative approach than traditional weight assessments like BMI.
DXA and BIS for muscle function
This study introduces new measures for assessing muscle function in sarcopenia using a combination of dual-energy X-ray absorptiometry (DXA) and bioimpedance spectroscopy (BIS). It proposes adjusting appendicular lean mass (ALM) and leg lean mass (LLM) by the ratio of extracellular to intracellular fluid, improving the prediction of muscle function, particularly jump power. The study finds that these novel measures, especially LLM corrected for fluid ratio, are more effective than traditional methods in predicting muscle function, suggesting their potential use in sarcopenia diagnosis.
Public Health Radiology
This study tested a deep learning-based model (DLBM) for improved automated segmentation of visceral and subcutaneous adipose tissues in CT scans. The DLBM, significantly faster and preferred by clinicians over the feature-based model (FBM), showed similar accuracy in predicting 5-year survival in colorectal cancer patients. Despite its efficiency and clinician preference, the DLBM's predictive performance for survival, measured using hazard ratios and area under the curve (AUC), was comparable to that of the FBM. This model demonstrates potential for efficient, automated CT scan analysis in clinical settings.
Learning Data Science
Professor Claudia Solis-Lemus and Ben Rush developed a website to introduce middle and high school students to data science and statistics using engaging media, R scripts, and relatable examples like dog breeds. The site offers an accessible approach to understanding data science concepts, focusing on real-world applications over mathematical formulas. It includes 11 lessons, humorous examples, RShiny apps, and links for further learning. The aim is to inspire young learners in applied mathematics fields by demonstrating the relevance of statistics and data science in decision-making and potential careers. The website plans to expand topics to data science and machine learning, making these subjects approachable even for students who might be math-averse.
Can’t get enough?
You can read Ben’s PhD Dissertation below or get a PDF copy here.